The Multimodal Music Tagging task 2012 strives to foster novel and creative multimodal approaches to learn relations between music items and semantic text labels. Attaching semantic labels to multimedia items is a very labor-intensive task if performed manually. Hence, methods that automatically assign a set of tags to a given piece of music are highly desired by the industry. Such auto-taggers further pave the way for various intelligent music retrieval applications, such as automated playlist generators or music recommendation systems. They also enable faceted browsing of music collections as well as semantic search.
In this task, participants will be given several sets of multimodal data related to music songs (see below). The aim is then to build an auto-tagger using some or all of the provided data sets. Including additional data sources is possible as well (e.g., music video clips, images of album covers, or song lyrics). Investigating which categories of tags (e.g., genres, styles, emotions, ...) can be learned well and which ones are more challenging is another relevant question that should be addressed.
Data:
We provide both a training set and a test set. The training set of 975 songs includes:
- metadata to identify the songs and the artists
- content descriptors computed using the MIRToolbox; at the moment MFCCs are provided, but participants are encouraged to compute any other descriptor by sending a request to musiclef (at) dei.unipd.it and providing the code (preferably Matlab code)
- collaborative tags extracted from last.fm (in the form of a list of terms)
- multilingual sets of web pages related to the artists/performers of the songs (both the complete pages and generic term weights are provided); 6 languages are covered
- the "ground truth" tags, made by experts in the field using a controlled dictionary of about 100 distinct tags
The test set of 380 songs includes all data mentioned above, except for the ground truth tags. Those will be made available to participants after submission of their results.
The goal of the task is to assign to each song in the test set one or more tags (taken from the controlled dictionary), using any combination of the above-mentioned data sources, from content-based features to contextual information. Performance will be assessed using standard IR measures such as precision, recall, and F1-measure.
Task organizers:
Nicola Orio, University of Padua, Italy
Geoffroy Peeters, Institut de Recherche et Coordination Acoustique/Musique Paris, France
Markus Schedl, Johannes Kepler University Linz, Austria
Cynthia Liem, Delft University of Technology, Netherlands
Note
This task is a "Brave New Task", which means that it will run as a closed task in 2012, with an eye to becoming a larger, open task in 2013. Participation is by invitation only. If you are interested in receiving an invitation, please write and email to musiclef (at) dei.unipd.it.
The task is made possible by a collaboration of projects including the "PROMISE Network of Excellence" funded by the 7th Framework Programme of the European Commission, Grant agreement no. 258191 Austrian Science Funds (FWF): P22856-N23 "Personalized Music Retrieval via Music Content, Music Context, and User Context".
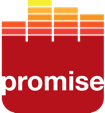