Register to participate in this challenge on the MediaEval 2016 registration site.
The task addresses the problem of the appearance and propagation of posts that share misleading multimedia content (images or video).
In the context of the task, different types of misleading use are considered (see Fig.1 below):
- Reposting of real multimedia, such as real photos from the past re-posted as being associated to a current event,
- Digitally manipulated multimedia,
- Synthetic multimedia, such as artworks or snapshots presented as real imagery.
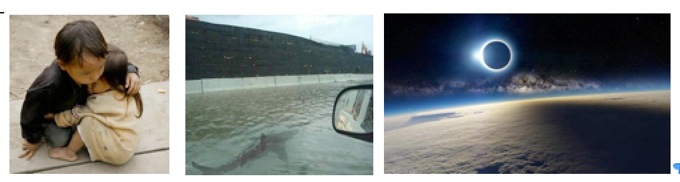
Figure 1. Examples of misleading image use: (i) reposting of real photo depicting two Vietnamese siblings as being captured during the Nepal 2015 earthquakes; (ii) spliced sharks on a photo captured during Hurricane Sandy in 2012; (iii) reposting of artwork as a photo from Solar Eclipse of March 20th, 2015.
Given a social media post, comprising a text component, an associated piece of multimedia (image/video) and a set of metadata originating from the social media platform, the task requires participants to return a decision (fake, real or unknown) on whether the information presented by this post sufficiently reflects the reality.
Background
This task aims to build and establish automatic ways to classify viral social media content propagating fake images or presenting real images in a false context. After a high impact event has taken place, a lot of controversial information goes viral on social media and investigation needs to be carried out to debunk it and decide whether the shared multimedia represents real information. As there is lack of publicly accessible tools for assessing the veracity of user-generated content, the task intends to aid news professionals, such as journalists, to verify their sources and fulfill the goals of journalism that imposes a strict code of faithfulness to reality and objectivity.
Why participate?
The task offers a cross-disciplinary playground involving the areas of multimedia indexing and search (e.g., near-duplicate detection), forensics (manipulation/tampering detection), Web mining and information extraction (trust-oriented feature extraction), machine learning (fake classification), information retrieval and crowdsourcing (trust assessment and labelling), while it is also a practical and attractive topic from a business point of view, for the sectors of news (journalists, editors), e-commerce (ratings, reviews), and more.
Hence, a number of researchers and practitioners from these areas would be interested in such a challenging task. In addition, it is worth mentioning that a number of EC-funded projects have recently been launched in this area. These include: REVEAL, the main driver behind this proposal), as well as Pheme, InVID, and MAVEN.
We encourage participants from diverse backgrounds to participate. These include image processing, Natural Language Processing, social network analysis, web mining and machine learning. Participants are encouraged to use any features for developing their system, either from the released ones or others developed by them. To allow a fair comparison there will be participant categories for image-only approaches, text only approaches and hybrid approaches.
Challenge awards will be provided for (1) best image forensics approach (2) best text analysis approach and (3) best hybrid approach. We want to encourage researchers to create image and/or text focused approaches and will recognize each type equally.
Data
The dataset will be a set of social media posts (e.g., Twitter) for which the social media identifiers will be shared along with the post text and some additional characteristics of the post. The associated multimedia item (image, video) will also be available. The dataset used for last year’s task is currently available on GitHub: https://github.com/MKLab-ITI/image-verification-corpus/tree/master/mediaeval2015 and part of it will be used for organizing the new training dataset. This year, we are also considering to provide Facebook and blog posts in addition to Twitter posts.
In order to cover diverse cases, the data provided will be formulated in a variety of languages. For each post, the original text, an auxiliary machined translated text and a language code will be provided to participants (i.e., translated English plus native language of post will be available).
We will also release three sets of features: text- and metadata-based features for the posts, user-based features for the user profiles of the post authors and multimedia forensic features for the images shared by the posts.
Lastly we will release for each event the claimed event location and time, as this would typically be known by a journalist when verifying a specific claim.
The ground truth of the training dataset will be available and will include a label that declares the veracity of the post: fake for the tweets with misleading content and real for the tweets with real content.
An explorative crowdsourcing task will be designed for the data collection with the aim to integrate different in nature data and keep in balance the posts per image case. This year's dataset will be extended by combining inputs from a crowdsourcing task with semi-automatic data expansion, filtering and validation methods.
Ground truth and evaluation
The task measures the accuracy of automated methods that distinguish between posts with multimedia content that share trustworthy (real) and those sharing misleading information (fake). Participants should predict the labels of the test set of data. Classic IR measures will be used in order to quantify performance using a per-event score averaging scheme. More concretely, F1 score is chosen for this task as a reliable and adequate metric of the performance of a classifier, even when a dataset is unbalanced.
The task will also ask participants to provide an explanation of their choice (plain text or a description of the way the decision has been taken), which will be used just for gaining useful insights and it will not be part of the evaluation.
Recommended reading
[1] Boididou, C., Papadopoulos, S., Kompatsiaris, Y., Schifferes, S., Newman, N. Challenges of computational verification in social multimedia. In Proceedings of the companion publication of the 23rd international conference on World wide web companion (WWW Companion '14), pp. 743-748
[2] Conotter, V., Dang-Nguyen, D.-T., Riegler, M., Boato, G., Larson, M. A Crowdsourced Data Set of Edited Images Online. In Proceedings of the 2014 International ACM Workshop on Crowdsourcing for Multimedia (CrowdMM '14). ACM, New York, NY, USA, 49-52
Task organizers
Christina Boididou, CERTH-ITI, Greece
Symeon Papadopoulos, CERTH-ITI, Greece
Stuart E. Middleton, University of Southhampton, UK
Giulia Boato, U. Trento, Italy
Duc-Tien Dang-Nguyen, U. Trento, Italy
Michael Riegler, Simula, Norway
Task schedule
6 May 2016: Development data released.
10 June 2016: Test data released.
9 Sept. 2016: Deadline for run submission.
23 Sept. 2016: Results returned.
30 Sept. 2016: Working notes paper deadline
20-21 Oct. 2016: MediaEval 2016 Workshop, Right after ACM MM 2016 in Amsterdam.
Acknowledgments
This task is supported by the REVEAL EC FP7 Project.



Check out the informative websites: http://revealproject.eu
and http://www.invid-project.eu