Task Results
- Task overview: [slides][video]
- Task results: [presentation videos]
- For all papers, see the MediaEval 2018 Working Notes Proceedings: http://ceur-ws.org/Vol-2283
Task description
Participants receive a set of images (representative of images shared on social media) and are required to enhance them. The enhancement should achieve two goals: (1) Protection: It must block the ability of an automatic pixel-based algorithm from correctly predicting the identify of the setting which the photo was taken (i.e., prevent automatic inference of the scene class) and (2) Appeal: It must make the image more beautiful or interesting from the point of view of the user (or at least not ruin the image from users’ point of view.)
Note about image enhancements: It may or may not be the case that the enhancement prevents a human viewer from correctly guessing the setting/location of the image. The task not focused on concealing sensitive information from humans, but rather from preventing automatic algorithms from carrying out large-scale inference. The reason is this: the ultimate goal of the task is to prevent large-scale location inference attacks, such as cybercasing. In other words, it should be impossible to automatically process a large set of images and pick out all the images that were taken in bedrooms or at vacation locations (which reflect that users sharing the images are not at home.)
Target group
We hope that this task attracts a wide range of participants who are concerned about privacy from computer scientists to artists and photographers. Within the field of computer science, people interested in machine learning, adversarial machine learning, computer graphics, privacy and computer vision will bind the task interesting.
Data
Data will be drawn from the Places365-Standard data set.
Ground truth and evaluation
Protection will be evaluated using the ground truth labels of the images (i.e., the correct class label). The protection score is the relative change in the prediction accuracy between the original, clean version of the test set images and the enhanced version produced by the participant. Note that we expect that accuracy to decrease after protection, but theoretically it is also possible that protection fails, and that it stays the same or even increases.
Appeal will be evaluated by an automatic aesthetics classification algorithm, and also by a set of human annotators, who will inspect the original image and the enhanced image, and give a rating to the acceptability of the change. The ratings will be collected on a 7-point scale, according to whether the enhancement is (1) very disturbing (2) noticeable and distracting, (3) noticeable and mildly distracting, (4) noticeable but not distracting, (5) not really noticeable, (6) noticeable and pleasant, (7) noticeable and either highly interesting or appealing.
Submissions will be ranked as follows: All approaches that achieve a protection score of at least 50% (50% reducing in the accuracy of the prediction) will be ranked in terms of their appeal score.
We will also recognize two other achievements: the highest appeal score (as long as evidence or an argument is presented that the approach has the future potential to reach protection score of 50%) and the highest protection score (as long as evidence or an argument is presented that the approach has the future potential to reach protection score of at least 5).
Recommended reading
Jaeyoung Choi, Martha Larson, Xinchao Li, Kevin Li, Gerald Friedland, and Alan Hanjalic. 2017. The Geo-Privacy Bonus of Popular Photo Enhancements. In Proceedings of the 2017 ACM on International Conference on Multimedia Retrieval (ICMR '17). ACM, New York, NY, USA, 84-92.
Ádám Erdélyi, Thomas Winkler and Bernhard Rinner. 2013. Serious Fun: Cartooning for Privacy Protection, In Proceedings of the MediaEval 2013 Multimedia Benchmark Workshop, Barcelona, Spain, October 18-19, 2013.
Gerald Friedland and Robin Sommer. 2010. Cybercasing the Joint: On the Privacy Implications of Geo-tagging. In Proceedings of the 5th USENIX Conference on Hot Topics in Security (HotSec’10). 1–8.
Task organizers
Simon Brugman, Zhuoran Liu, Martha Larson, with help from Zhengyu Zhao
Radboud University
Task schedule
15 September: Data release
12 October: Submission (of protected images)
19 October: Return of results
26 October: Finalization of working notes paper
Acknowledgements
NWO TTW Open Mind
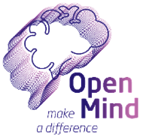