This page was updated on 10 September 2014 to reflect the final form of the task.
The MediaEval 2014 Crowdsorting task addresses the classification of multimedia comments. The classifier makes use of labels that have been collected from the crowd. Because these labels are collected under typical crowdsourcing conditions, they are characterized by a certain level of noise. The tasks asks participants to carry out consensus computation: i.e., given a set of noisy labels for a given items, to predict a single `correct’ label.
Optionally, participants can predict labels for the items by combining the input from the crowd (i.e., human computation) with automatic computation (i.e., text and/or audio signal analysis).
The ultimate goal of the task, is to improve systems and algorithms that use timed comments. Timed comments are comments that are made by users at a particular point in time within a multimedia stream. In this task, we concentrate on music on the SoundCloud audio sharing platform. This screen capture from SoundCloud shows timed-comments as they are displayed in the interface.
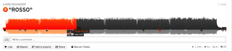
The comments are depicted along the time-line of the sound. (Note that SoundCloud refers to the audio that users upload to its platform as “sounds”, and we also adopt this terminology for this task, rather than referring to “songs” or “pieces of music”). Each comment is displayed as the icon of the commenting user positioned at the time-point at which that user added a comment. When the sound is played, the comments open and can be read at the moment during the sound at which the user originally added them. In this screen capture, it can also be seen that a sound on SoundCloud is associated with additional textual information, e.g., a title (in this case “Rosso”) and the name of the uploading artist (Ilario Schanzer). For a better understanding of SoundCloud sounds and timed-comments, visit the sound depicted above directly on SoundCloud https://soundcloud.com/ilario-schanzer/rosso
We are interested in timed-comments because of their potential to improve users’ access to music and to support them in discovering new music. Specifically, timed-comments mention aspects of music that are difficult to derive from the signal, and may be useful to calculate song-to-song similarity needed to improve sound recommendation. The fact that the comments are related to a certain time point is important because it allows us to derive continuous information over time from a sound (an aspect also explored by the Emotion in Music Task). Timed-comments are potentially very helpful for supporting listeners in finding specific points of interest within a sound, or deciding whether they want to listen to a sound, since they allow users to jump-in and listen to specific moments, without listening to the sound end-to-end.
This year the data set is built on the basis of timed comments that users have contributed to techno music. We focus on segments of music in which a user has indicated in a timed comment that it contains a drop.
Target group
Researchers in the area of human computation or social media analysis (e.g,. text classification). The task can also be addressed by incorporating music analysis.
Data
The data set was released on the Open Science Framework: https://osf.io/h92g8
Ground truth and evaluation
The ground truth used to evaluate the task consists of a set of ‘high fidelity labels’ (i.e., high quality votes on the appropriate category for each comment) that are collected from expert annotators.
The official evaluation metric will be the F1 score, weighted to take into account the relative size of the classes.
Recommended reading
[1] Loni, B., Cheung, L.Y., Riegler, M., Bozzon, A., Gottlieb, L., Larson, M. Fashion 10000: An Enriched Social Image Dataset for Fashion and Clothing. In Proceedings of MMSys Multimedia Systems Conference. Scottsdale, Arizona, USA, 2014.
[2] Loni, B., Larson, M., Bozzon, A., Gottlieb, L. Crowdsourcing for Social Multimedia at MediaEval 2013: Challenges, Data Set, and Evaluation. In Proceedings of the MediaEval 2013 Multimedia Benchmark Workshop, CEUR-WS.org, 1043, ISSN: 1613-0073. Barcelona, Spain, 2013.
[3] Sheshadri, A., Lease, M. SQUARE: A Benchmark for Research on Computing Crowd Consensus. In Proceedings of AAAI HCOMP Conference on Human Computation and Crowdsourcing. Palm Springs, California, USA, 2013.
[4] Vliegendhart, R., Loni, B., Larson, M., Hanjalic, A. How Do We Deep-link?: Leveraging User-contributed Time-links for Non-linear Video Access. In Proceedings of ACM International Conference on Multimedia. ACM, Barcelona, Spain, 2013, 517-520.
Task organizers
Karthik Yadati, Delft University of Technology, Netherlands
Martha Larson, Delft University of Technology, Netherlands
Task auxiliaries:
Pavala S.N. Chandrasekaran Ayyanathan, Delft University of Technology, Netherlands
Mohammad Soleymani, University of Geneva, Switzerland
Task schedule
1 September: Data release
28 September: Working notes paper deadline
Note that this task is a "Brave New Task" in 2014. If you sign up for this task, you will be asked to keep in particularly close touch with the task organizers concerning the task goals and the task timeline.
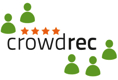

