Task Results
Task overview: [Slides] [Presentation video]
Participant results: [Playlist of all presentation videos]
Task Description
The goal of our task is to understand how genre classification can explore and address the subjective and culturally-dependent nature of genre categories. Traditionally genre classification is performed using a single source of ground truth with broad genre categories as class labels. In contrast, this task is aimed at exploring how to explore and combine multiple sources of annotations, each of which are more detailed. Each source has a different genre class space, providing an opportunity to analyze the problem of music genre recognition from new perspectives and with the potential of reducing evaluation bias.
Full information about the task can be found on the AcousticBrainz Genre Task 2017 github page.
Task organizers
Dmitry Bogdanov, Music Technology Group, Universitat Pompeu Fabra, Spain (first.last @upf.edu)
Alastair Porter, Music Technology Group, Universitat Pompeu Fabra, Spain (first.last @upf.edu)
Julián Urbano, Music Technology Group, Universitat Pompeu Fabra, Spain
Hendrik Schreiber, Tagtraum Industries Incorporated, USA
Task schedule
11 May: Development/Test data release (updated)
14 August: Run submission
21 August: Results returned to participants
28 August: Working notes paper deadline
13-15 Sept: MediaEval Workshop in Dublin
Acknowledgements
AcousticBrainz and Audio Commons


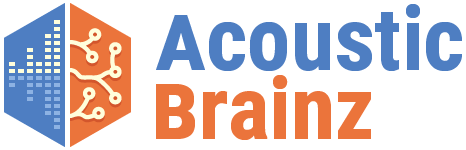
This research has received funding from the European Union’s Horizon 2020 research and innovation programme under grant agreement No 688382.